From 1998-2002, I was at the MIT CADlab working on my Ph.D., focusing on how life cycle assessment (LCA) can be wisely used in design for the environment. Early design stages are critical in shaping the environmental performance of a product over its life cycle, yet they create particular challenges for environmental assessment. I focused my research on this question: “How can product design teams quickly evaluate and trade off competing product concepts using the scarce information available at early conceptual stages?“
My exploration of this topic was informed by a core practical requirement: environmental evaluation techniques must be operable within the constraints of real-world product development and provide credible, timely information that is sufficient for decision-making.
Why early design stages? Typically 75% of the manufacturing cost is committed by the end of conceptual phase in the design process. This means that decisions made after this point in the design process can determine only 25% of the product’s manufacturing cost. Likewise, the environmental performance of a product is largely locked into the product early in the design process, when critical decisions are made on key product attributes such as materials, energy requirements, and longevity, which ultimately determine the life cycle performance of the product.
Design effect in manufacturing cost
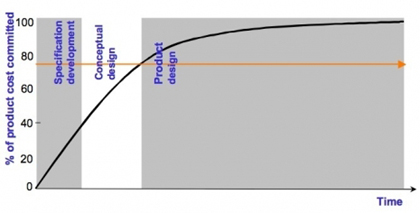
Adapted from “The Mechanical Design Process”, David G. Ullman, McGraw-Hill 1992.
Environmental ‘lock-in’ by design activities over the product development process and life cycle
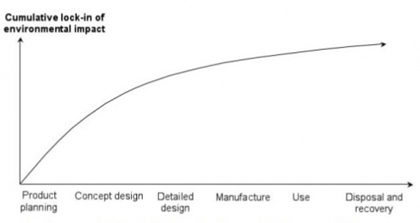
Adapted from “Design + Environment – a Global Guide to Designing Greener Goods”, Lewis, H., Gertsakis, J., Grant, T., Morelli, N. and Sweatman, A., New York: Greenleaf Publishing 2001.
Life cycle assessment (LCA) is a powerful decision support tool that systematically and quantitatively fuels a holistic, systems view of products, with great potential to both guide the selection of design options and provide a spectrum of useful insights on opportunities for improvement and innovation. But detailed LCA is costly, time-consuming, and data intensive. Early design stages lack the detailed information needed for thorough assessments and require quick decisions on diverse, numerous and loosely-defined product concepts, making the early use of detailed LCA impractical.
This set of ideas and challenges framed my thesis work on ‘streamlining’ LCA to bring life cycle thinking and quantitative analysis into early conceptual product design. Going ‘wild’, I looked to discover new ways to quickly generate, use and re-use LCA data and knowledge at early design stages, ultimately exploring new territories in the land of artificial intelligence. The ‘learning surrogate LCA’ concept was created and successfully tested.
Here’s how the learning surrogate LCA works:
- An artificial neural network (ANN) is trained on product attributes and environmental performance data from pre-existing full LCA studies.
- The product design team queries the trained artificial model with high-level product concept descriptors (e.g. material types and amount, recycled content, type of energy source, in-use power consumption, type of fuel and fuel consumption, life time drive distance, driver behavior!).
- The team quickly obtains an approximate environmental performance assessment (e.g. life cycle energy consumption, global warming potential, acidification potential, waste index) for a new or re-designed product concept, without requiring a new LCA model, and under the guidance of environmental experts who train, validate and maintain the ANN-based LCA models. The product design team can then use the predicted environmental performance, along with key performance measures from other models, in trade-off analyses and concept selection.
Proof-of-concept testing showed that the ANN-based learning surrogate LCA models were able to: (a) match detailed LCA results within the accuracy of typical LCA studies, (b) predict relative differences of distinct product concepts, and (c) correctly predict and generalize trends associated with changes for a given product concept. As a data-driven modeling approach, the learning surrogate LCA needs data – lots of product LCA data. The increasing demand and supply of product LCA studies will provide this needed data, hopefully sooner rather than later!
Learning surrogate LCA concept
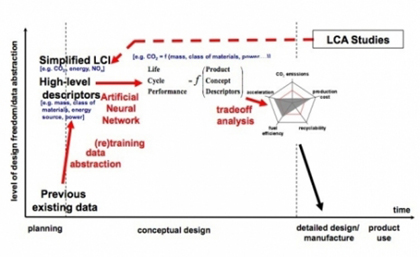
Adapted from "Approximate Life-Cycle Assessment of Product Concepts Using Learning Systems", Inês Sousa, Ph.D. Thesis, Cambridge, MA: Massachusetts Institute of Technology, 2002.
Learn more about this work: “ Approximate Life-Cycle Assessment of Product Concepts using Learning Systems” published in the MIT Journal of Industrial Ecology in 2000.
Sustainable Minds: It’s my new adventurous journey that logically followed. It started at one of Lauralee Alben’s Sea Change Design Workshops where I met Terry, my Co-founder. Read her blog post next for the next installment:
Part 2: The genesis of Sustainable Minds – Things happen in threes
Also read Part 3: The genesis of Sustainable Minds – How we met Philip White and Okala
Image credits:
Fig 1. Design Effect in Manufacturing Cost
credit: Adapted from “The Mechanical Design Process”, David G. Ullman, McGraw-Hill 1992.
Fig 2. Environmental ‘lock-in’ by design activities over the product development process and life cycle
credit: Adapted from “Design + Environment – a Global Guide to Designing Greener Goods”, Lewis, H., Gertsakis, J., Grant, T., Morelli, N. and Sweatman, A., New York: Greenleaf Publishing 2001.
Fig 3. Learning surrogate LCA concept
credit: Adapted from "Approximate Life-Cycle Assessment of Product Concepts Using Learning Systems", Inês Sousa, Ph.D Thesis, Cambridge, MA: Massachusetts Institute of Technology, 2002.